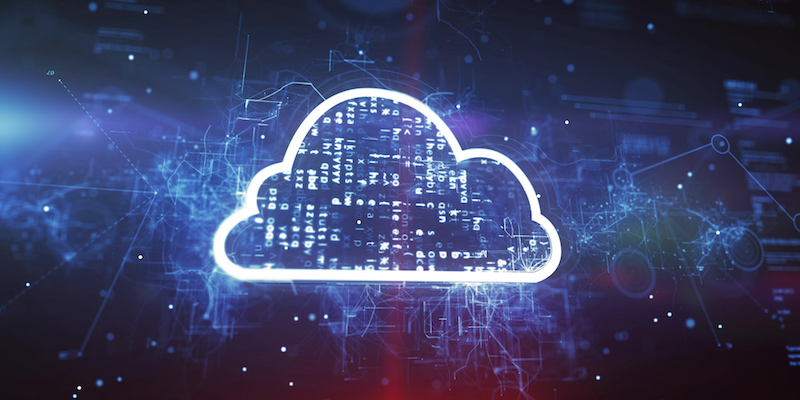
In the world of high performance computing (HPC) speed is king. Like highly tuned racing cars, the latest hardware and software offerings are compared in terms of FLOPS (floating-point operations per second), communications latency (in microseconds) and bandwidth (gigabits per second) and clock speed (gigahertz) – though the latter is now giving way to the core-count, or number of CPU cores within each device.
All of this speed is designed to make computing tasks run faster and thereby increase the turnaround time and profitability of the end user. In an environment where large in-house computers are bought every 4-5 years and capital budgets have recurring line-item values, these figures make perfect sense for the end user.
In the modern world of cloud supercomputing, the cost-benefit of performance hardware can be more finely assessed. Is it worth paying a premium for a high-specification machine when a cheaper one would be more cost effective and achieve the same task? How well does the configuration of any given offering match the task required?
Zenotech provides HPC on-demand services to its customers in aerospace, automotive, civil and renewable engineering via the web-based portal EPIC. End users used to challenge the use of on-demand cloud-based HPC on the basis of security or data access, but technology advances plus the overwhelming business case is now very hard to refute.
To illustrate the saving that can be made via on-demand resourcing, consider the task of running a computational fluid dynamics (CFD) job with a pressing 24-hour engineering deadline. CFD tasks are major consumers of compute power and are often the limiting factor in achieving rapid responses for simulation-based design.
CFD Simulation of flow around a wing mirror
Suppose that the job requires a computer with over 2000 cores and that this peak requirement has been used to size the in-house purchase of a conventional supercomputing cluster. Taking into account the purchase price, overheads, infrastructure and power, such a machine will provide computing resource – if fully utilised – at about £0.08 per core-hour. Note that this is an estimate, but it will be typical for most end users. Specialist organisations may be able to reduce this cost closer to £0.05 per core but hour but very few will be able to achieve less than this.
This particular task takes 16 hours, and 2176 cores, so the total internal cost is £2,785. If the internal machine were block-booked for the full 24 hours of course – the cost would arguably be higher because the reduced utilisation rate inflates the core hour price by 50%.
Now consider the option of accessing a similar resource online. Such a resource would cost at most about the same as the in-house cluster, but it is likely to be much cheaper. This is true in the first instance because only the time used will be paid for – so the effective utilisation rate is 100%. Secondly the core hour rate will probably be lower than the internal cost because of economies of scale. Cloud HPC providers buy hardware in significant volume and so enjoy larger discounts, plus they spread the overheads (including labour) over a larger estate so the core-hour price bears a smaller overhead loading. Even if the online hardware is marginally less well tuned for a specific application, the low prices and massive availability will compensate.
The Amazon Web Services (AWS) spot pricing market – where end users bid for resource – is a very cost effective way to access large computers for Zenotech customers. When there is availability (which is most of the time), spot instances are up to 90% discounted when compared to their reserved instance counterparts. This means that our previous computing task now costs £279! How a company decides to leverage this advantage is its own business – either by completing a more exhaustive analysis with a greater number of runs for the same price, or by running larger jobs to deliver higher fidelity.
Launching an AWS based HPC cluster with EPIC
Imagine now that we are in the business of running both large, highly accurate jobs (against which requirement we have sized the in-house cluster and paid extra for a high-speed interconnecting switch) plus optimisation and design space characterisation studies. The latter are based on very large numbers of smaller job runs, and so do not need the expensive switches and can be run on smaller, cheaper machines. Turnaround time is no longer a function of raw monolithic HPC performance, but volume.
A typical design space search might require up to a million small tasks to be run. These independent tasks span a set of design parameters of interest and the analysis tasks return a set of data about how each variant has performed. Sometimes these responses are used to refine a set of design space queries and repeat the operation – each new generation of solutions providing a step towards a better engineering insight into the product being developed.
If we assume that each task requires 8 CPU cores for 1 hour, then the time required on the existing in-house system is 6 months, at a cost of £639k. The same task run on-demand could in the extreme case only require 1 hour as all tasks could be completed independently. However if we spread the job out over 48 hours in order to use spot pricing at the lowest hourly rate the job would cost just £64k and take 2 days.
This is a compelling business argument for the use of cloud resources, especially for high performance computing. Even though Amazon AWS usually does have newer hardware than our engineering customers (as they buy more frequently) the turnaround time that can be delivered by massive volume and selecting the right infrastructure for the job – when you need it – is the new way of delivering speed.
For more on AWS HPC see https://aws.amazon.com/hpc/
For more on EPIC see https://epic.zenotech.com