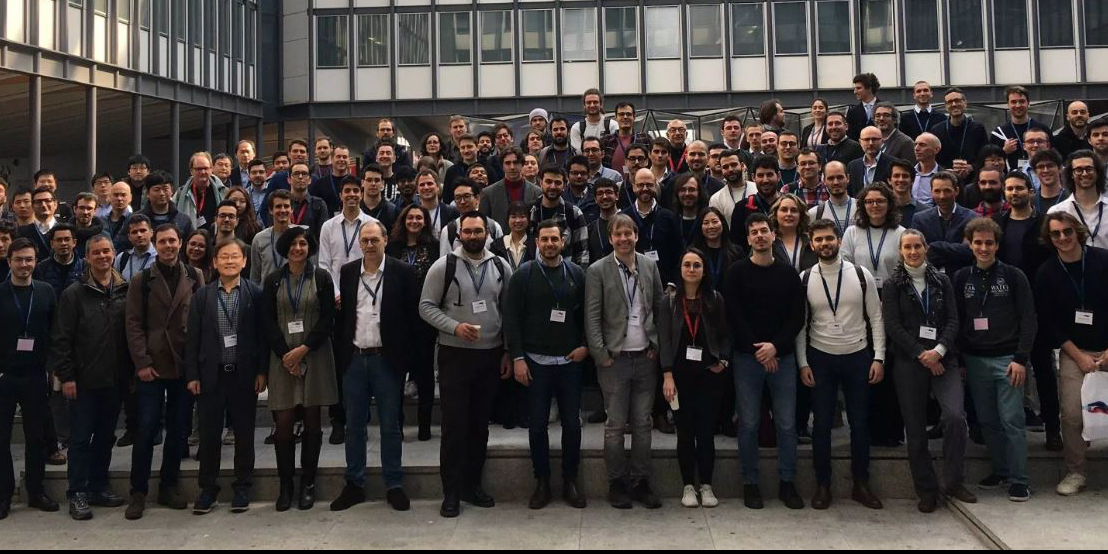
The field of fluid dynamics and machine learning is rapidly emerging as an interesting area of innovation. However, directly applying existing machine learning tools, often developed for unrelated fields, may be inadequate for the intricacies of fluid dynamics and physics problems.
That’s why the ERCOFTAC (European Research Community of Flow, Turbulence and Combustion) Special Interest Group, Machine Learning for Fluid Dynamics is making such a valuable contribution to the field.
David Standingford, Zenotech’s CEO and co-founder, participates in this SIG bringing an industrial viewpoint, and recently, our colleague Constantinos Vagianos spoke at the Machine Learning for Fluid Dynamics workshop.
After the workshop’s success, we’re delighted to put the spotlight on the co-ordinator of the Special Interest Group, Paola Cinnella of the Sorbonne. Professor Cinnella’s research focuses on numerical methods for fluid dynamics and their application to the analysis and design of compressible flows.
Read her full interview below:
You’re the co-ordinator of the ERCOFTAC Special Interest Group 54 in Machine Learning for Fluid Dynamics. Why did you want to set up this SIG?
Machine Learning is transforming all fields of knowledge, and an exponential growth of such applications to fluid flow simulation and analysis has been observed in recent years. However, the contributions are inconsistent, and there is a lack of common test cases and benchmarks to assess and compare different approaches. The ambition of the SIG is to bring together this scattered and diverse community to discuss pressing issues, collect data, and set common practices, test cases, and benchmarks.
The Machine Learning for Fluid Dynamics workshop was a huge success. What were the highlights of the workshop?
The workshop attracted 160 registered participants globally with participants from Europe, the US, Canada, Japan, and Korea. We had five insightful plenary talks:
- Bruno Desprès, for the Jacques Louis Lyons Institute, who talked about the mathematical foundations of machine learning;
- Romit Maulik, from Penn State University, who presented recent results on machine-learning augmented fluid models and reinforcement learning;
- Nils Thuerey, from Technical University Munich, with experience in both fluid dynamics and computer graphics, discussed the properties of neural differential equations and their training;
- Heinz Pitsch, from Technical University Aachen, presented advances in turbulent combustion modeling using generative machine learning;
- Emmanuel De Bézenac, from ETH Zurich, talked about neural operators for solving parametrized flow problems.
The workshop was so valuable as it involved not only researchers from the fluid dynamics community applying Machine Learning to fluid problems but also applied mathematicians and computer scientists with an interest in applications of Machine Learning to the solution of partial differential equations and fluid flow problems.
Why is this topic gaining so much attention?
Fluid flow problems are very nonlinear and multiscale. Although a whole hierarchy of models, ranging from semi-analytical to massively parallel scale-resolving codes, exist to study them, it remains very difficult to achieve high-quality solutions without using brute-force HPC methods. Machine learning has a tremendous potential to speed up/emulate costly simulations, “augment” cheap low-fidelity models using high-fidelity data, compressing data, fusing data of various origins, supersampling coarse solutions, extracting flow structures, and learning control strategies.
However, a naïve application of existing machine learning tools, initially developed for computer vision or other purely statistical tasks, is insufficient. Joint efforts from fluid dynamicists, computer scientists, and applied mathematicians are needed to develop Machine Learning methods for physics problems. That is what SIG 54 is trying to do.
What are the key developments to keep an eye on in this area?
Neural operators are a promising approach for learning mappings between function spaces, e.g., for learning relationships between a family of geometries/boundary conditions and the associated flow fields.
Data-driven turbulence modeling has also made a lot of progress and is helping to renew research on Reynolds-Averaged approaches that have been almost abandoned for more than a decade.
Finally, Machine Learning combining low-fidelity and scale-resolving models into a multi-fidelity supermodel is extremely attractive for shape optimization or uncertainty quantification tasks.
What other related projects are you involved in?
I am participating in a European project named SciFiTurbo that aims to use Machine Learning and scale-resolving simulations to design the next generation of ultra-efficient aeronautical engines
I am also setting a chair on Machine Learning for Science at Sorbonne University, in collaboration with Prof. Patrick Gallinari, a renowned expert in deep learning from Sorbonne’s computer science department. Our goal will be to investigate generative AI and how to learn foundation models (i.e. large models such as ChatGPT) to describe and predict fluid flow systems.
Can participants expect to see another Machine Learning Workshop soon?
I am happy to answer, yes! Given the success of our inaugural workshop, ERCOFTAC has decided to have an annual conference series on Machine Learning for Fluids. The next one will be a joint ERCOFTAC/Euromech event in 2025. Stay tuned for more information!
Thanks to Paola for the interview and we look forward to the next Machine Learning for Fluid Dynamics Workshop next year.
Discover more in David Standingford’s thought leadership article on bridging the gap between Machine Learning, AI and Machine Learning.